Unlocking (Google's view of) the future of generative AI
Unlocking (Google's view of) the future of generative AI
A deep dive into Google Cloud Masterclass on Data and Generative (2023 Edition)
Dear BlackCube members and fellow tech enthusiasts,
Today, we present you with a treasure trove of insights from Google’s recent Masterclass held on September 15, 2023. The event was a masterful exploration of how to leverage data and generative AI and its expansive possibilities, which seamlessly aligns with BlackCube’s ethos of harmonizing human creativity and AI precision. Let's delve in, shall we?
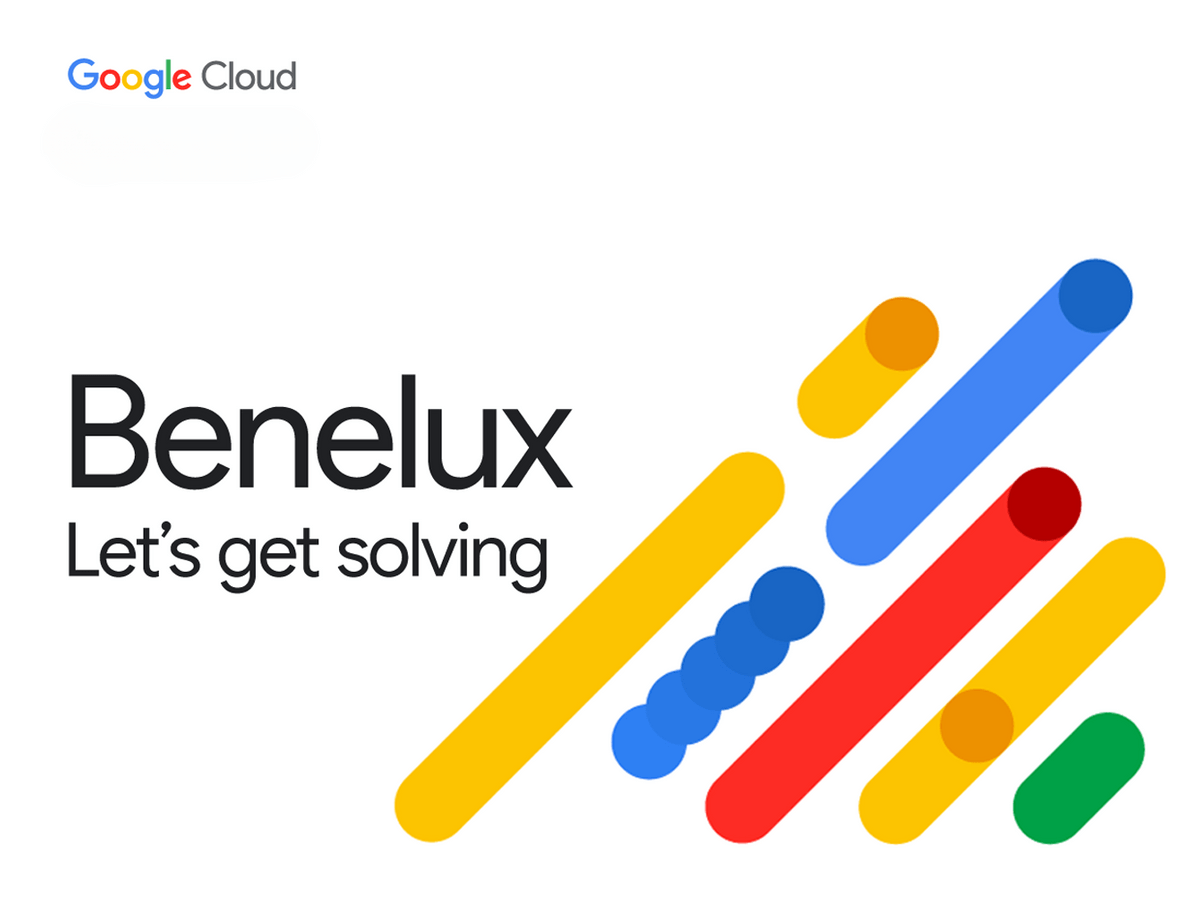
🎙 Our guides
- Rokesh Jankie Customer Engineering Manager at Google Cloud
- Vidhi Jain from Google Cloud
- Sebastian from Weaviate
🤓 Introduction & Context
The event was part of the recent Google Cloud Summit Benelux held on October 12-13, 2023. Speakers from Google and other industry leaders presented, focusing on a multitude of areas from the technological aspects to the practical applications of Generative AI. The summit itself was organized around two days: Day 1 - business-centric, and Day 2 - focused on hacks.
🌀 The Universe of Generative AI
📌 Machine Learning vs. Deep Learning
The event opened with the assertion that machine learning is deep learning. "Attention is all you need" said the first speaker. In simpler terms, attention mechanisms help deep learning models focus on relevant features, creating a more accurate and nuanced model.
Some interesting definitions:
- Machine learning is a broader field that encompasses various techniques and methods for training machines to learn from data
- Deep learning, on the other hand, is a specialized subfield of machine learning that employs neural networks with three or more layers. These neural networks attempt to simulate the behavior of the human brain—albeit far from matching its ability—to "learn" from large amounts of data. While all deep learning is machine learning, not all machine learning is deep learning.
The concept behind "Attention is all you need"
This is particularly influential in the realm of deep learning. Originating from a seminal paper, this phrase encapsulates the importance of attention mechanisms in neural networks. These mechanisms allow models to focus on specific parts of the input data relevant to the task at hand, much like how humans pay attention to particular aspects of an object or situation when learning or making decisions. In computational terms, attention mechanisms weigh the importance of different parts of the input and then allocate computational resources accordingly. This enables deep learning models to achieve more accurate and nuanced outputs, especially in complex tasks like language translation, image recognition, and even generative art.
By integrating attention mechanisms, deep learning models can streamline their "thought processes", prioritizing the most pertinent information. This results in not only improved performance but also more efficient use of computational resources. Attention mechanisms have thus become a cornerstone in the evolution of deep learning, marking a significant advance in the field’s capabilities.
📌 What is generative AI?
Generative AI models learn patterns through training data. Then, using that acquired knowledge, they can predict what comes next in a given context. Think of it as AI's creative essence—something we at BlackCube have been translating into generative art and NFTs.
Some interesting definitions:
- Generative capabilities: The ability of AI to create new, meaningful data based on the patterns it has learned.
- Intelligence: The measure of a model's ability to make accurate and logical decisions.
- Multimodal sensing: The utilization of multiple data types (like text, image, audio) by an AI model.
📌 Risk mitigation
Google reassures that proper data governance prevents IP leakage. Moreover, using correct datasets and effective prompting can minimize the risk of AI "hallucinations" or errors.
(IP) leakage underlines the critical need for robust security measures in AI deployments. IP leakage can have disastrous consequences, not only impacting the organization but also potentially violating legal obligations concerning sensitive or proprietary data. To forestall such situations, a comprehensive data governance framework should be put in place. This includes robust encryption protocols, access control measures, and regular audits to ensure that the data is handled in a secure and compliant manner.
The mention of using "correct datasets and effective prompting" to minimize AI "hallucinations" or errors addresses another vital aspect of risk mitigation. In the context of generative AI, "hallucinations" refer to instances where the AI generates data that is not just incorrect but also completely unrelated or nonsensical. These can arise from biases in the training data, ineffective model architecture, or poor prompting.
- Correct datasets: Utilizing well-curated, unbiased, and representative datasets is a proactive measure to ensure that the generative models are trained on a solid foundation. The quality of the dataset will directly influence the output, thus making it imperative to exercise due diligence in dataset preparation and selection.
- Effective prompting: The prompt serves as a contextual guide for the AI model, steering it toward generating data that aligns closely with the intended objective. A poorly designed prompt can lead to ambiguous or erratic outputs. Therefore, prompt design should be an iterative process that is continuously refined based on model performance and objectives.
By adhering to these guidelines, organizations can significantly mitigate the inherent risks associated with deploying generative AI models.
📌 Applications galore
Generative AI offers immense possibilities in conversational AI, enterprise search, and customer experiences—areas that align with BlackCube’s suite of unique AI tools and community platform!
🛠 Tools & Technologies
📌 Magic editor & Generative AI solutions for ads
Google's Magic Editor is an intelligent platform that integrates seamlessly with Google's cloud technologies. It allows businesses and creators to employ generative AI algorithms to produce dynamic and contextually relevant advertisements. Utilizing machine learning models, the Magic Editor can automate A/B testing, target audience segmentation, and even generate ad copy or visual elements. This results in highly personalized and effective marketing campaigns that are capable of adapting in real-time to audience interactions and feedback.
📌 Duet AI
Duet AI is a premium service that brings the power of AI to Google Workspace and Gmail, enabling a plethora of productivity enhancements. Imagine auto-scheduling features that learn your habits, AI-driven content suggestions within Google Docs, and smart tagging of emails based on their priority and content. Duet AI is like having a virtual assistant that understands your workflow, helps manage your tasks, and even preemptively troubleshoots issues before they escalate, thereby streamlining your operations and saving valuable time.
📌 Vertex AI
A powerful platform designed with scalability in mind, Vertex AI is tailored for developers, data scientists, and enterprises. It provides a one-stop-shop for creating, deploying, and maintaining AI models. Unlike traditional platforms, it offers flexibility in terms of pre-built solutions for common use-cases, as well as the ability to customize models for specific needs. Resources such as Generative AI Studio and Model Garden provide in-depth tutorials, pre-trained models, and libraries to accelerate the process of implementing generative AI solutions.
📌 Powered by nVidia
Google’s partnership with nVidia is a strategic alignment that significantly boosts the computational capabilities of its generative AI initiatives. nVidia's state-of-the-art GPUs are designed for high-speed, parallel processing, making them well-suited for the heavy computational needs of deep learning and generative models. This results in faster training times, more efficient model deployment, and real-time data processing capabilities, thereby significantly reducing the time-to-market for AI solutions.
🛡 The pillars & Best practices
📌 Prompt engineering
One of the most crucial factors for efficient human-AI collaboration is the design of the prompt. It serves as the interface through which humans interact with AI systems and thus, it should be finely crafted. Here's a breakdown of its components:
- Persona: This refers to the tone and voice of the AI. Whether it's formal, informal, or specialized for specific industries, the persona helps to set the user's expectations and fosters a more fluid interaction.
- Context: Providing constraints and details ensures that the AI's responses are aligned with the user's needs. For example, if you're using AI for customer service, the context could include guidelines for handling frequently asked questions or complaints.
- Task / Steps: Clearly outlining what needs to be done is pivotal. Whether it's summarizing an article, generating code, or creating a piece of art, the task specification allows the AI to focus its computational power efficiently.
- Examples: These guide the output by showing the AI model what is expected of it. They can range from previous successful interactions to a portfolio of case studies.
- Follow-up: For more complex tasks or ongoing interactions, follow-up steps can be designed to guide the AI through multi-stage processes, ensuring seamless and effective completion of tasks.
📌 Risk mitigation
Proactively managing risks in AI deployments is vital. This includes:
- Prompt Design: Carefully designed prompts reduce the likelihood of generating inappropriate or nonsensical output.
- Fine-tuning: Periodically revising and adjusting the AI model ensures it evolves in line with changes in data, user behavior, and external factors.
- Regulatory Preparation: Before deploying any AI model, it's essential to understand the legal and ethical constraints, particularly around data privacy and intellectual property.
- Safety Tests: These should be rigorously conducted to ensure the model's responses are within acceptable ethical and legal boundaries.
- Monitoring: Even after deployment, constant monitoring is necessary to catch any anomalies or errors, making real-time adjustments as necessary.
📌 Responsible AI guides
In line with the growing awareness of the ethical implications of AI, Google offers a suite of guides focused on Responsible AI (RAI). These guides help users understand best practices around fairness, interpretability, and the ethical use of AI. They offer frameworks and methodologies to identify, measure, and mitigate potential risks, thereby fostering responsible AI usage.
Each of these elements forms the bedrock of a robust and responsible generative AI practice. Careful attention to each can vastly improve the reliability, efficiency, and ethical standing of AI deployments.
That's a wrap. Remember, the future isn't just to be predicted; it's to be co-created with AI. Until next time, keep exploring, keep experimenting.
Andrea
Founder of BlackCube